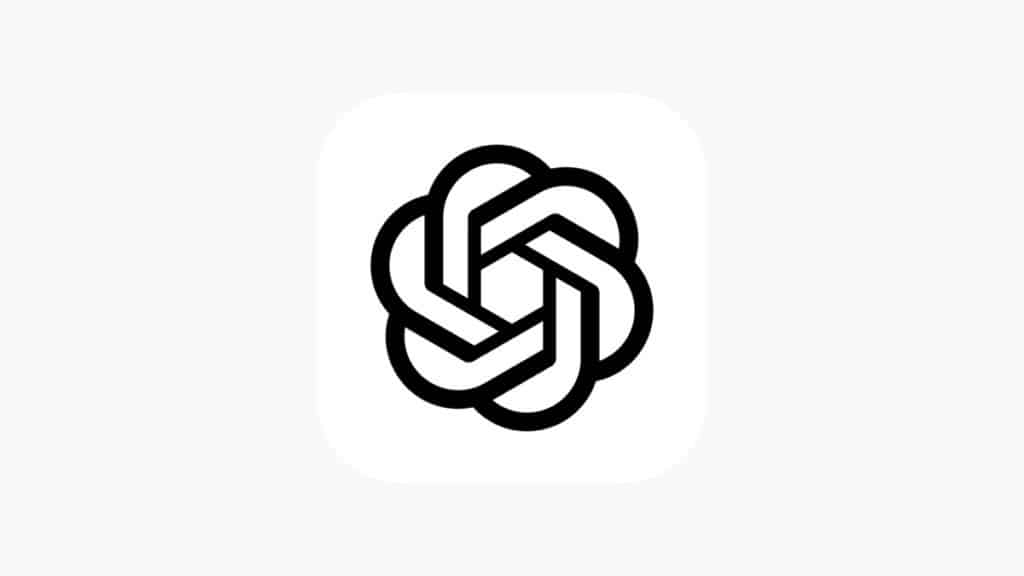
OpenAI has announced that its AI classifier, a project meant to help readers distinguish between human-written and AI-generated text, is no longer available due to its low rate of accuracy. “In our evaluations on a ‘challenge set’ of English texts, our classifier correctly identifies 26% of AI-written text (true positives) as ‘likely AI-written,’ while incorrectly labeling human-written text as AI-written 9% of the time (false positives),” OpenAI explained in its original post, which included six limitations that implied the classifier was never going to be fully reliable to begin with. OpenAI says that it is looking at feedback to create a better alternative, alongside other classifiers for determining whether audio or images are AI-generated.
Our classifier is a language model fine-tuned on a dataset of pairs of human-written text and AI-written text on the same topic. We collected this dataset from a variety of sources that we believe to be written by humans, such as the pretraining data and human demonstrations on prompts submitted to InstructGPT. We divided each text into a prompt and a response. On these prompts we generated responses from a variety of different language models trained by us and other organizations. For our web app, we adjust the confidence threshold to keep the false positive rate low; in other words, we only mark text as likely AI-written if the classifier is very confident.
OpenAI AI Classifier Limitations
- The classifier is very unreliable on short texts (below 1,000 characters). Even longer texts are sometimes incorrectly labeled by the classifier.
- Sometimes human-written text will be incorrectly but confidently labeled as AI-written by our classifier.
- We recommend using the classifier only for English text. It performs significantly worse in other languages and it is unreliable on code.
- Text that is very predictable cannot be reliably identified. For example, it is impossible to predict whether a list of the first 1,000 prime numbers was written by AI or humans, because the correct answer is always the same.
- AI-written text can be edited to evade the classifier. Classifiers like ours can be updated and retrained based on successful attacks, but it is unclear whether detection has an advantage in the long-term.
- Classifiers based on neural networks are known to be poorly calibrated outside of their training data. For inputs that are very different from text in our training set, the classifier is sometimes extremely confident in a wrong prediction.