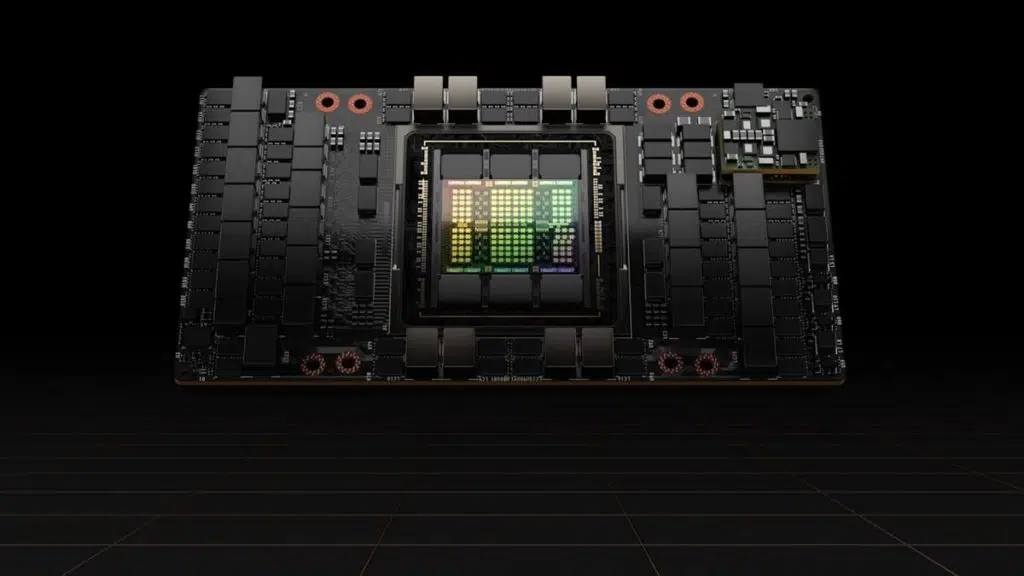
How is Huang’s Law doing these days? Phenomenal, it seems, as NVIDIA has shared a new article that describes how it’s been able to improve single GPU performance on AI inference by 1000x, and it did so in just 10 years’ time. According to a chart that was shown off during a recent talk by NVIDIA Chief Scientist Bill Dally, the story originally began with the Tesla K20X—a GPU Accelerator that was released back in 2013—but its performance has now been completely eclipsed by more recent products that include the H100, NVIDIA’s series of Hopper-powered GPUs that can deliver up to 68 teraFLOPS of performance. NVIDIA’s biggest gain was achieved from “finding simpler ways to represent the numbers computers use to make their calculations,” Dally explained.
It’s an astounding increase that IEEE Spectrum was the first to dub “Huang’s Law” after NVIDIA founder and CEO Jensen Huang. The label was later popularized by a column in the Wall Street Journal.
The advance was a response to the equally phenomenal rise of large language models used for generative AI that are growing by an order of magnitude every year.
In his talk, Dally detailed the elements that drove the 1,000x gain. The largest of all, a sixteen-fold gain, came from finding simpler ways to represent the numbers computers use to make their calculations.
The latest NVIDIA Hopper architecture with its Transformer Engine uses a dynamic mix of eight- and 16-bit floating point and integer math. It’s tailored to the needs of today’s generative AI models. Dally detailed both the performance gains and the energy savings the new math delivers.